Exploring Multi-modal Neural Scene Representations
With Applications on Thermal
Imaging
-
Mert Özer*
FAU Erlangen-Nürnberg -
Maximilian Weiherer*
FAU Erlangen-Nürnberg -
Martin Hundhausen
FAU Erlangen-Nürnberg -
Bernhard Egger
FAU Erlangen-Nürnberg
* indicates equal contribution.
RGB
RGB
RGB
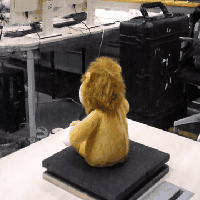
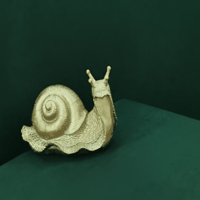
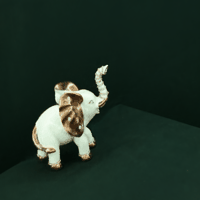
Thermal
IR
Depth
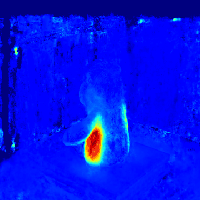
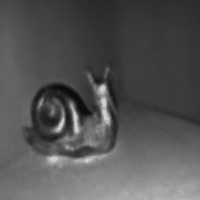
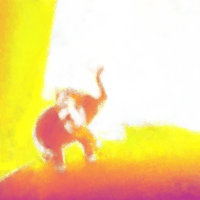
Abstract
Neural Radiance Fields (NeRFs) quickly evolved as the new de-facto standard for the task of novel view synthesis when trained on a set of RGB images. In this paper, we conduct a comprehensive evaluation of neural scene representations, such as NeRFs, in the context of multimodal learning. Specifically, we present four different strategies of how to incorporate a second modality, other than RGB, into NeRFs: (1) training from scratch independently on both modalities; (2) pre-training on RGB and fine-tuning on the second modality; (3) adding a second branch; and (4) adding a separate component to predict (color) values of the additional modality. We chose thermal imaging as second modality since it strongly differs from RGB in terms of radiosity, making it challenging to integrate into neural scene representations. For the evaluation of the proposed strategies, we captured a new publicly available multi-view dataset, ThermalMix, consisting of six common objects and about 360 RGB and thermal images in total. We employ cross-modality calibration prior to data capturing, leading to high-quality alignments between RGB and thermal images. Our findings reveal that adding a second branch to NeRF performs best for novel view synthesis on thermal images while also yielding compelling results on RGB. Finally, we also show that our analysis generalizes to other modalities, including near-infrared images and depth maps.
Overview
Summary of evaluated strategies for multi-modal learning.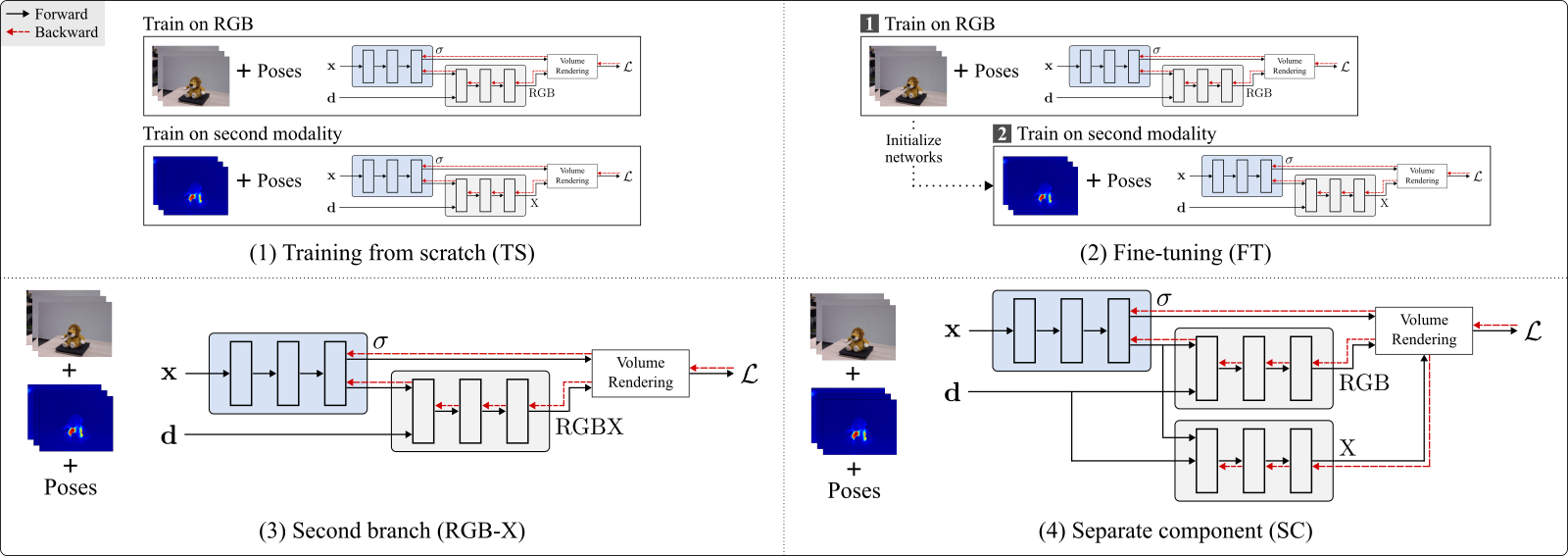
Overview of the four strategies that we compare within this work. In the first strategy (TS), we
train a NeRF-like base model
(Instant-NGP [23] in our case) from scratch, separately for RGB and the second modality. In the
second strategy (FT), we first pre-train
our base model on RGB data and then fine-tune on images of the second modality. While RGB-X adds a
second branch, strategy four
(SC) adds an extra network to predict (color) values of the additional modality. Note that RGB-X and
SC yield a single, multi-modal scene
representation, whereas TS and FT always result in two separate models, one for each modality.
Results of RGB-t for Thermal(proposed)
RGB information enhances the reconstruction of thermal images significantly. Especially for 360°-inward scenes.
(click and drag to swipe)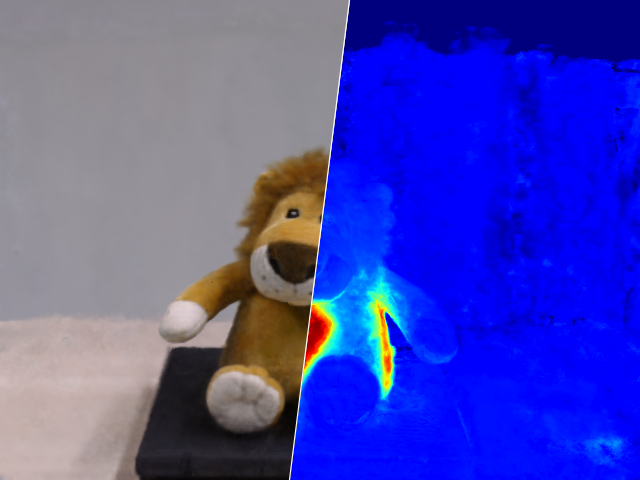
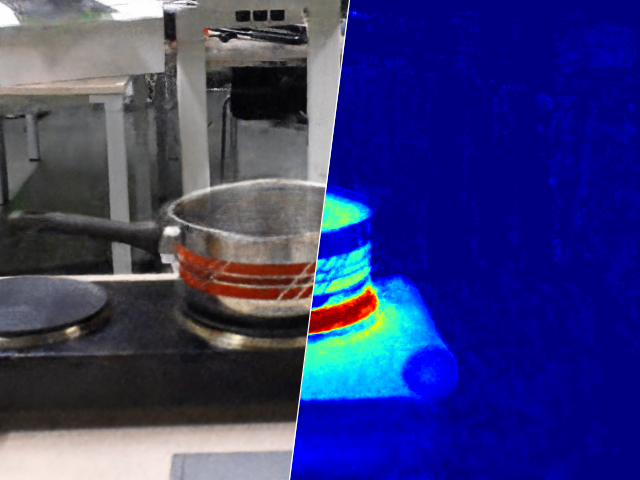
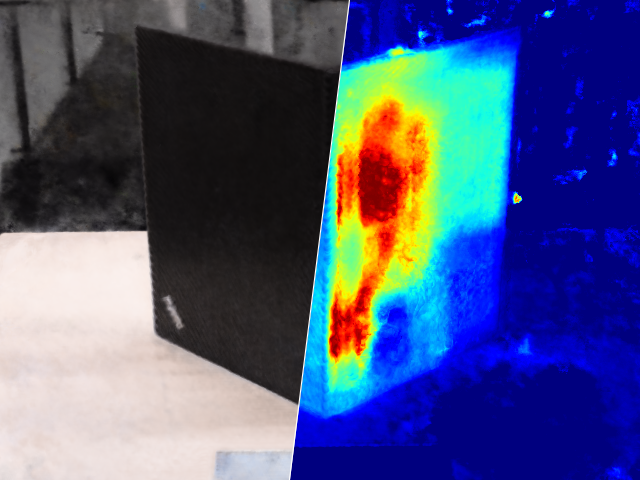
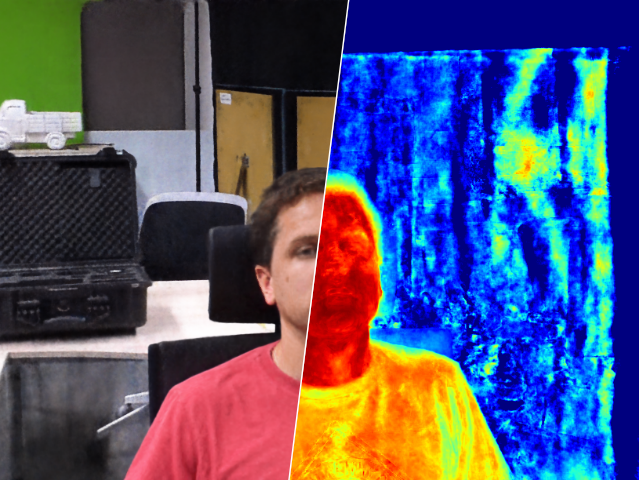
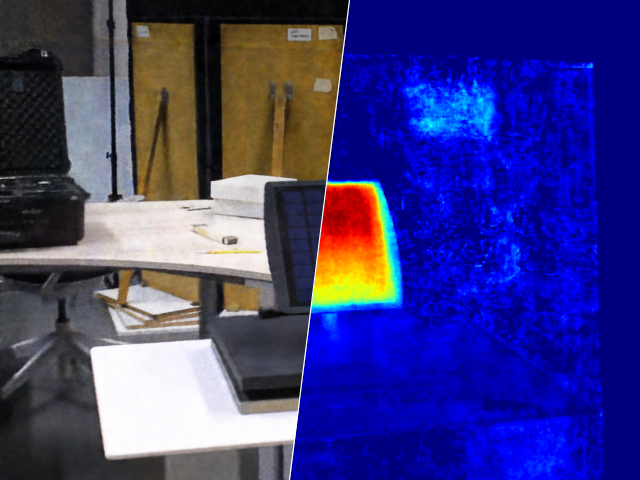
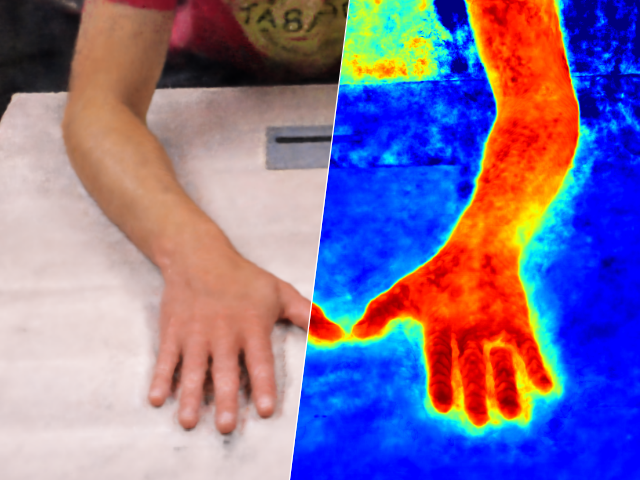
Results of training from scratch for Thermal(TS)(baseline)
TS forms the baselines for both RGB and Thermal reconstruction.
(click and drag to swipe)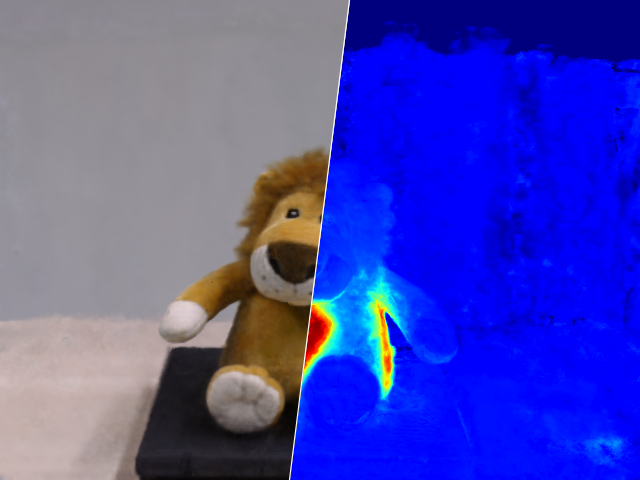
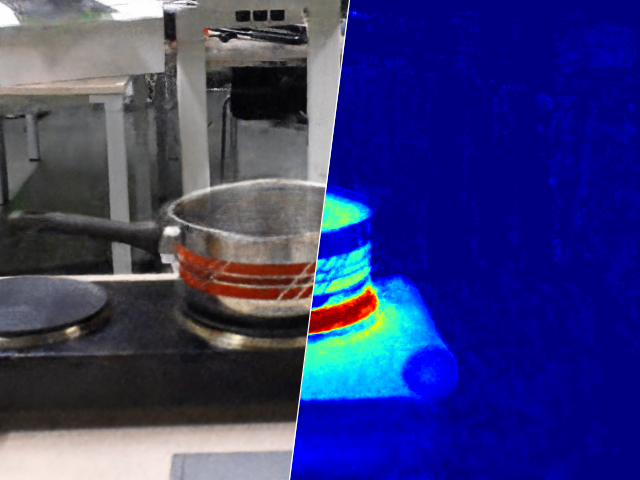
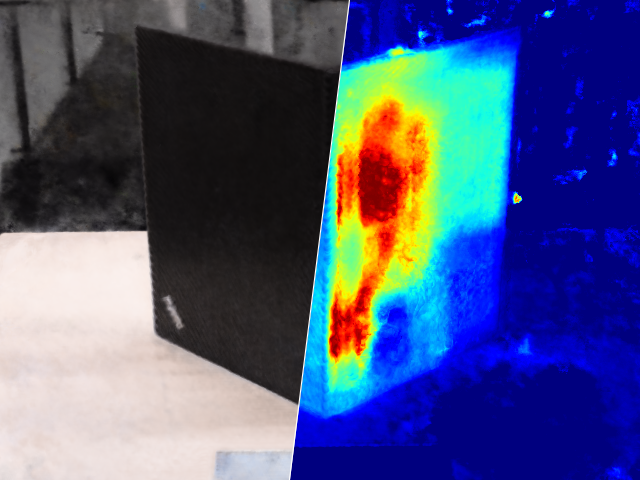
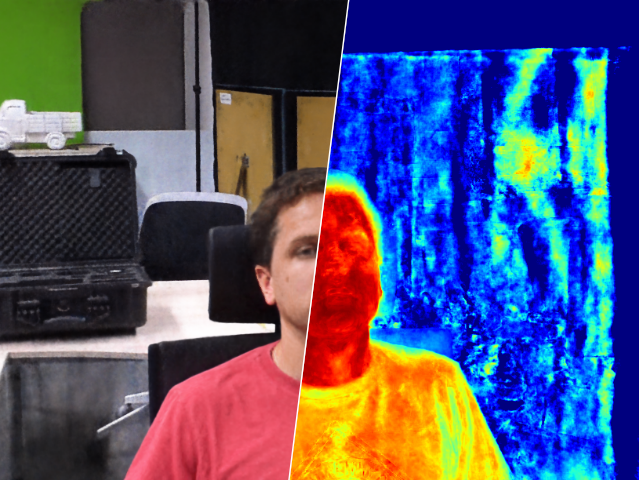
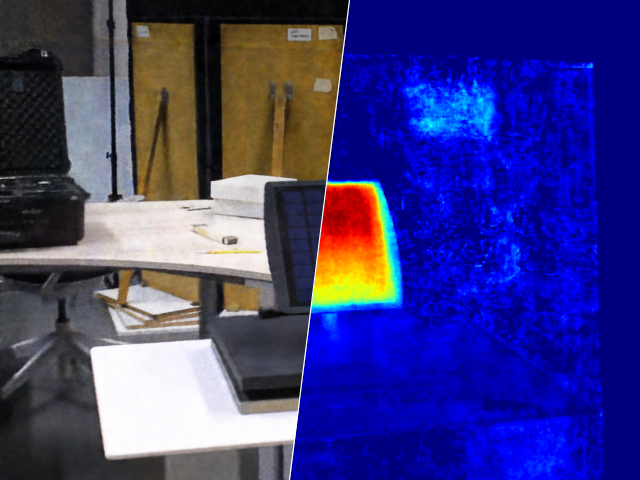
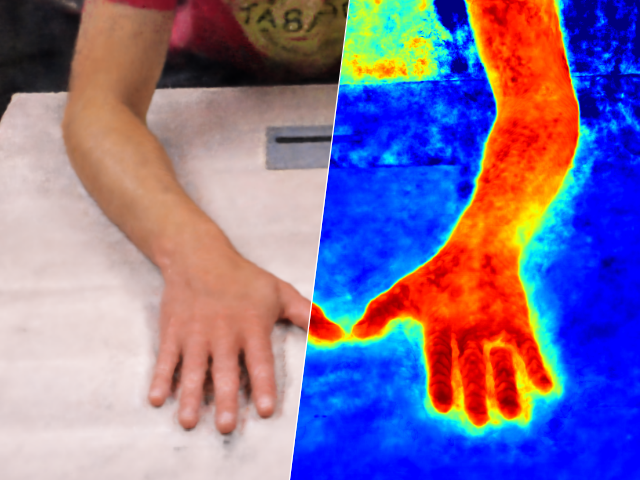
Results per view
Hover over the images to see the temperatures (click and drag to swipe)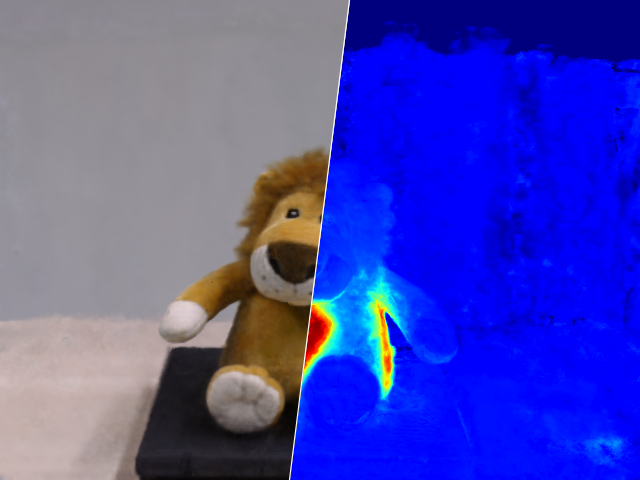
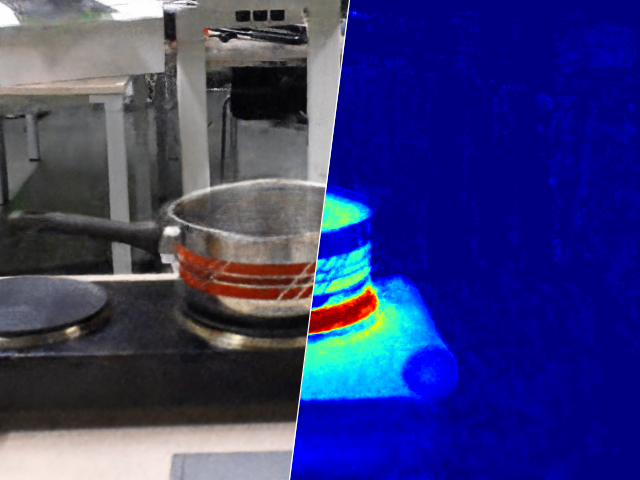
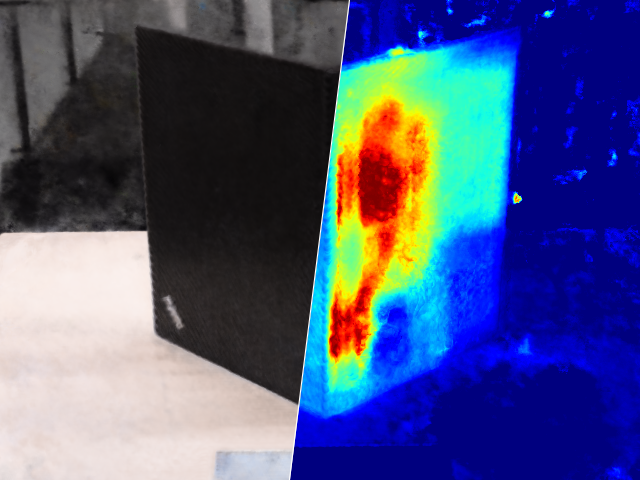
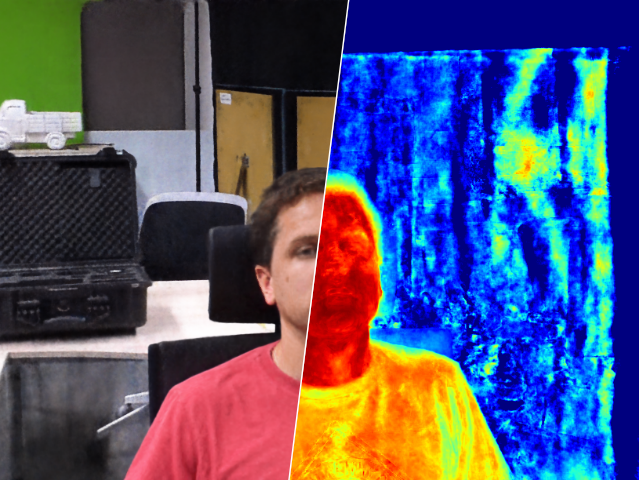
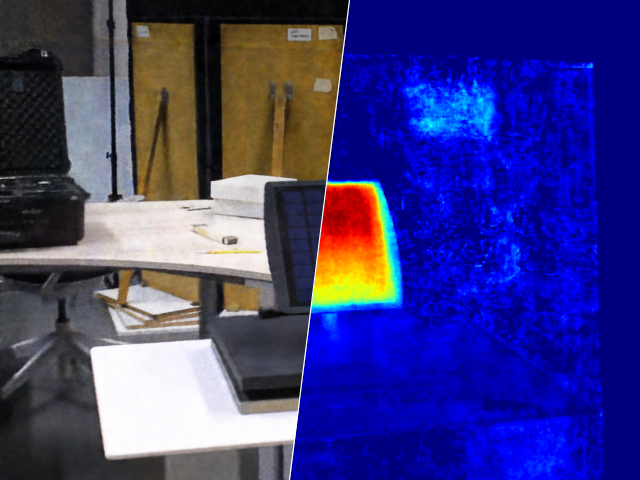
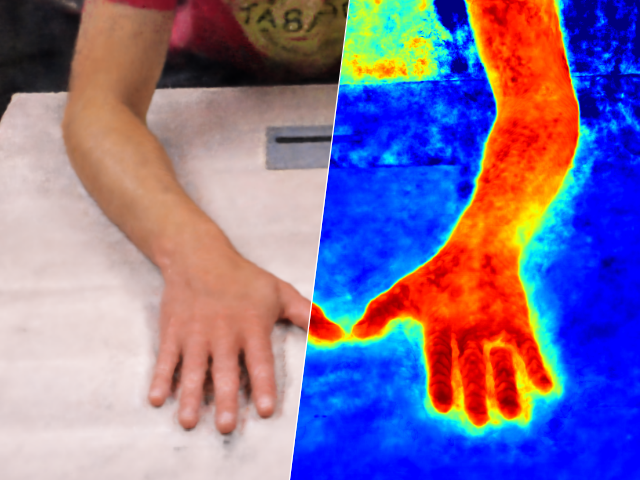
RGB-X for IR
(click and drag to swipe)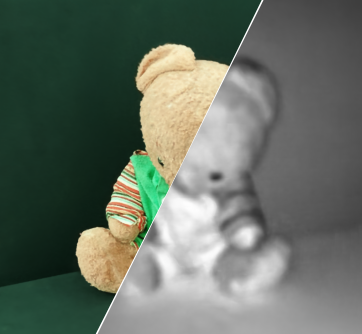
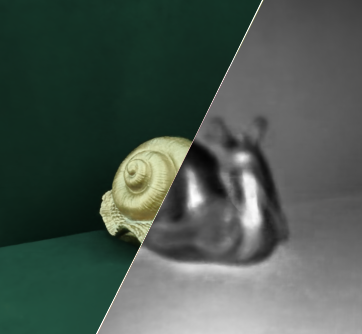
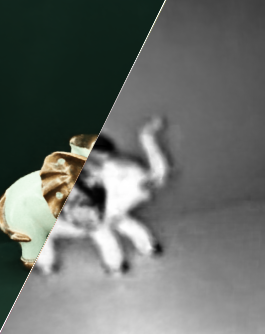
RGB-X for Depth
(click and drag to swipe)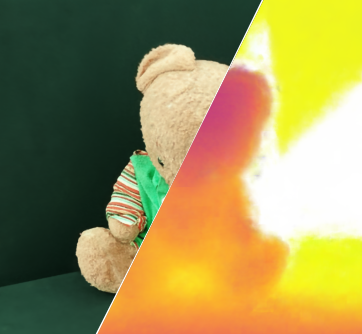
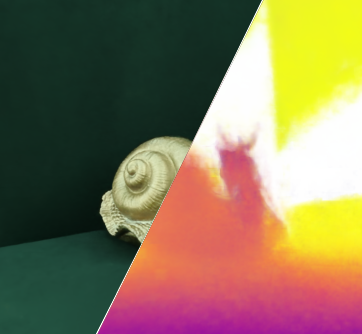
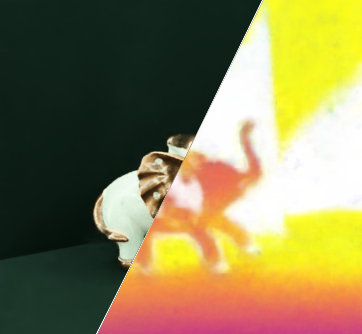
Acknowledgements
We would like to thank Ian Marius Peters, Bernd Doll and Oleksandr Mashkov for valuable discussions and access to the thermal camera.This work was funded by the German Federal Ministry of Education and Research (BMBF), FKZ: 01IS22082 (IRRW). The authors are responsible for the content of this publication.
The website template was adapted from Zip-NeRF, who borrowed from Michaël Gharbi and Ref-NeRF. Image sliders are from BakedSDF.
References
[Mildenhall 2020] Mildenhall, B., Srinivasan, P. P., Tancik, M., Barron, J. T., Ramamoorthi, R., & Ng, R. 2020. "NeRF: Representing Scenes as Neural Radiance Fields for View Synthesis." In Proceedings of the European Conference on Computer Vision (ECCV).
[Müller 2022] Müller, T., Evans, A., Schied, C. & Keller, A., 2022. "Instant neural graphics primitives with a multiresolution hash encoding." ACM Transactions on Graphics (ToG) 41.4 (2022): 1-15.